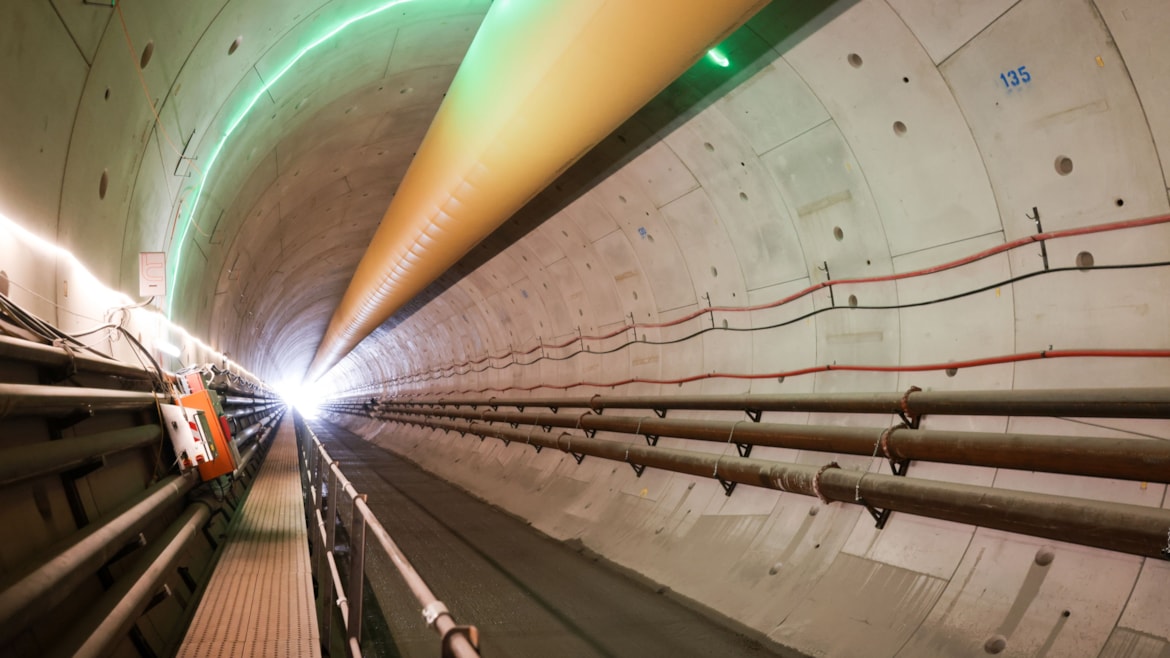
How do you successfully deploy machine learning in infrastructure? Tom Bartley of Mind Foundry, a startup being incubated by HS2, walks through the eight steps.
In January, HS2, in partnership with Connected Places Catapult and the Department for Transport, announced the latest cohort in its seventh Innovation Accelerator programme. It selected six UK-based technology firms to develop new railway technology to help build the line and underpin future train service reliability.
Initiatives like the HS2 accelerator are becoming increasingly common as those responsible for managing and maintaining our civil infrastructure explore opportunities to augment their work with AI or machine learning (ML). But how exactly does this work in practice? What‘s going on behind the scenes? And how would you deploy AI or ML to the infrastructure you manage?
Mind Foundry is one of the companies seeking to harness its AI-enabled technology to aid HS2 engineers to monitor the condition of the line’s structures and equipment for performance changes. This will underpin the railway’s reliability by enabling a ‘predict and prevent’ approach to renewals and replacements. Behind all of its technology deployments is a clear method that enables infrastructure managers and civil engineers to build, deploy, maintain, and scale AI effectively and responsibly.
Laying the foundations for an AI model
Deploying AI in an organisation is a continuous process, from the moment the use case is explored to when a model is retired. Each stage requires bringing together people with different expertise to ensure a successfully built and deployed AI system. But it all starts with two questions: what problem are you solving, and what would best solve it?
Step 1: Identifying the problem and defining the use case
The first stage of the AI lifecycle is to determine whether AI is the right tool to help solve your identified problem. This will usually be led by a digital transformation manager, product manager, project manager, and/or solutions engineer. In other words, the experts responsible for progressing the project throughout the AI lifecycle. This step provides the space to better explore and understand the business problem and the pain points to be solved.
In the case of HS2, this would involve asking how maintenance risks can be reduced by using AI and ML. One answer would be to enable a ‘predict-and-prevent’ approach to managing railway maintenance efficiently. This would mitigate structural risks and minimise potential losses by predicting potential issues early.
Step 2: Risk-and-responsibility assessment
With a clear understanding of the problem, developing an AI risk register document is essential for addressing ethical and operational risks. This document tracks potential AI challenges, mitigation strategies and assigned responsibilities. Responsible AI deployment is critical, particularly in infrastructure like HS2, where decisions impact public safety and the ultimate goal is to support human decision-making, not replace it.
Step 3: Data collection and preparation
The next step is data. Quality data is the backbone of useful AI models. Vast and complex infrastructure projects like HS2 provide ideal environments for AI-driven data analysis, with an abundance of sources to draw from.
Data scientists work alongside engineers to gather, clean and structure data, ensuring that features extracted from datasets can be applied further down the line. Feature engineering (the process of preparing raw data for ML models) then refines these inputs.
For example, when monitoring the maintenance of railway lines, custom-built AI algorithms can analyse these unique datasets to identify patterns and trends in the lines themselves, or the surrounding environment, that could indicate future risks.
Step 4: Feasibility exploration
Feasibility testing determines whether AI solutions align with technical requirements before full-scale deployment. In this stage, technology partners will rigorously assess the viability of AI solutions to ensure they deliver tangible operational benefits.
Step 5: Model training, evaluation and validation
Once feasibility has been established, it’s time to build and train the model. ML scientists train models using historical and current data, refining them through iterative testing to optimise accuracy.
Step 6: Real-world model deployment
Once trained, AI models can be deployed within infrastructure systems. Depending on operational needs, deployment may occur in the cloud or on edge devices. For example, rural railway assets may require drones that can inspect sites and process data without constant connectivity.
Step 7: Human-AI interface and experience
Deployment often goes hand in hand with user experience (UX) and the key UX challenge when designing for deployment is about building trust in the collaboration between humans and AI. From the technologist’s point of view, this is about interpretability, transparency and explainability. But from the civil engineer’s perspective, it is about being able to understand model predictions with clear indicators of accuracy and uncertainty. Additionally, interfaces must clearly frame model accuracy to help users make informed decisions, as most predictions are not 100% accurate.
Human-AI interaction is about how users engage with the model output, enabling the user to correct and provide feedback to the model and allowing it to improve continuously over time.
In high-stakes applications like civil infrastructure projects, ensuring that decision-making remains human-led and creating intuitive interfaces to facilitate the collaboration between humans and responsible AI is of utmost importance.
Step 8: Maintaining model performance
For a railway line that will need to adapt to environmental and operational changes, the ability for models to expand and develop in line with such changes is crucial.
Studies indicate that 91% of AI models degrade over time due to model drift (changes in the relationship between input and output data) and data drift (shifts in the data distribution).
Continuous meta learning reduces the risks of model degradation and drift, enabling AI systems to self-adapt based on real-world changes and human feedback. As a result, any models being deployed to help maintain infrastructure should be equipped with the ability to learn and evolve.
Building the future with AI
As many built assets near critical degradation thresholds, AI-driven predictive maintenance will be crucial for prolonging their lifespan. Infrastructure managers can ensure safe, reliable and efficient AI adoption by following a structured AI lifecycle.
However, successful AI adoption requires more than enthusiasm: it demands a nuanced understanding of different ML models and their best applications. While AI offers transformative potential, unlocking its full benefits hinges on targeted deployment, rigorous oversight and continued adaptation.
For those in infrastructure, the first steps are about understanding what you are dealing with and where it can take you. HS2’s structured approach to AI adoption provides a valuable blueprint for other infrastructure projects, demonstrating how AI can enhance operational efficiency while ensuring human oversight and accountability.
By investing in AI literacy, establishing governance frameworks, and initiating pilot projects, infrastructure managers can integrate AI responsibly and build a more resilient, data-driven environment.
Don’t miss out on BIM, information management and digital construction news: sign up to receive the BIMplus newsletter.