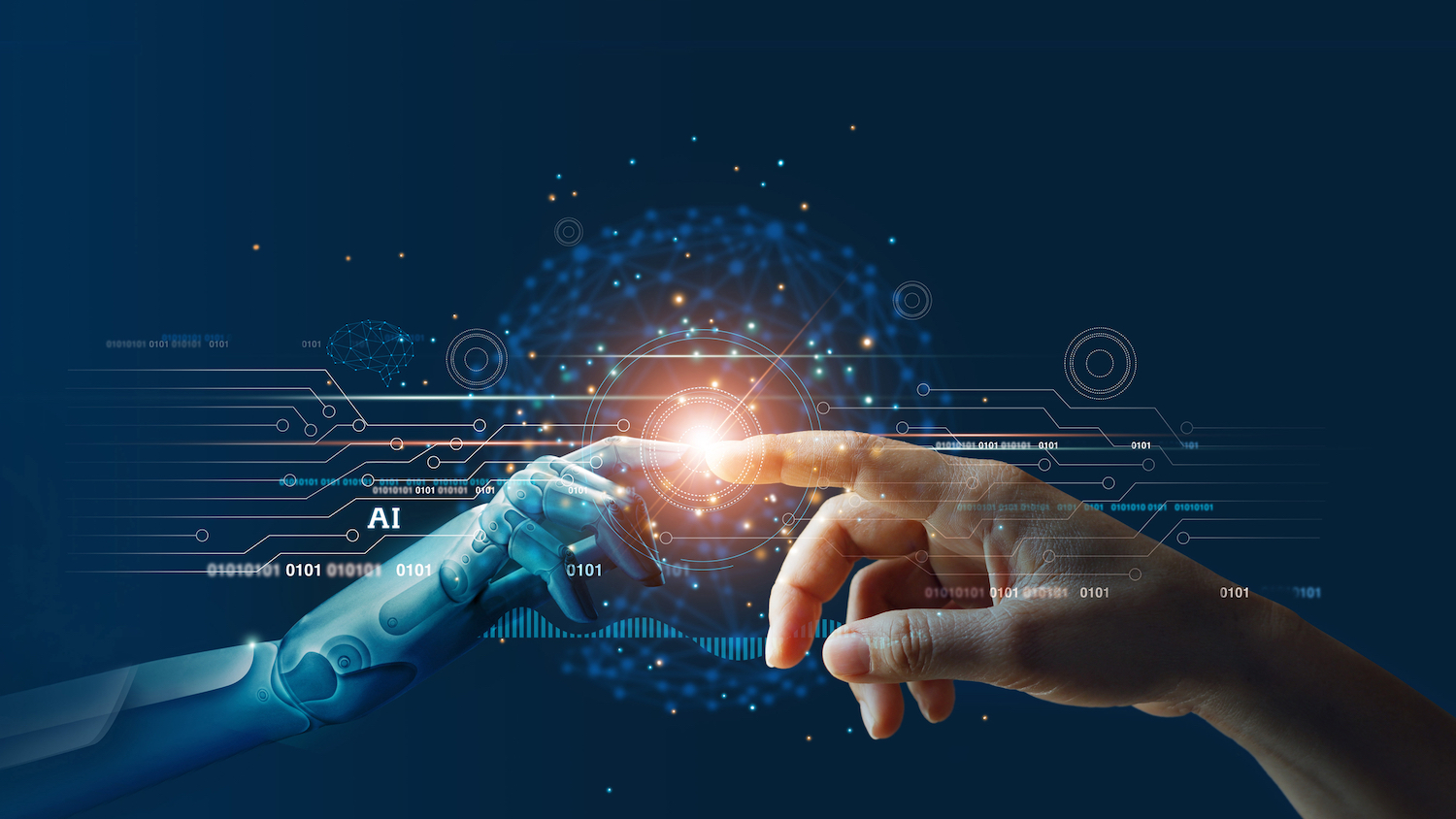
The CIOB AI playbook, launched this week, proposes a 12-step strategy for implementing AI into an organisation and its workflows.
The CIOB AI playbook aims to support construction companies of all sizes in the use of the technology, in particular SMEs. It offers ways to evaluate AI’s effectiveness, while also considering matters such as ethics, cyber security and data protection.
There is also a checklist to support new entrants, early adopters and experienced AI practitioners in making decisions about their use of the technology.
The 12-step strategy takes a common sense and practical approach, acknowledging the known issues with AI:
- Determine what can AI do – potential uses (based on types of AI).
- Perform a gap analysis – what do we need that AI can help with?
- What skillsets are needed in-house and outsourced?
- What existing off-the-shelf solutions and tools can be used?
- What uses need custom solutions?
- What types of AI do we want to use?
- What bespoke tasks and dashboards will be created for those bespoke uses?
- What data is needed for them, what do we have, what’s missing, how can that affect results, and what algorithms to use?
- How can we assess deliverables quality/performance – are there any KPIs?
- What are their risks/legal implications? Who is liable for them?
- How often will the AI need to be updated?
- How to integrate them with existing in-house and supply chain systems?
Data quality
The Playbook also includes a chapter written by Vicki Reynolds, chief technology officer at Catalyst, that highlights the importance of data quality in the deployment of AI. She flags six key areas:
- Accuracy and reliability – AI models learn patterns and make predictions based on the data they are trained on. If the data is accurate and reliable, the model is more likely to produce accurate and reliable results. Poor-quality data can lead to incorrect conclusions and unreliable predictions.
- Cost efficiency – Poor-quality data can lead to inaccurate predictions, which can result in costly errors and inefficiencies. Investing in high-quality data from the beginning can save resources in the long run by reducing the need for model retraining and correction of errors.
- Generalisations – AI models are often designed to generalise patterns from training data to make new predictions and include those in future outputs. High-quality data ensures that the model learns relevant and representative patterns, allowing it to generalise well in new situations.
- Bias mitigation – Biases present in training data can be learned and perpetuated by AI. Data taken from sources such as the internet (web scraping) are particularly susceptible to bias. Data should not be skewed toward specific groups or perspectives.
- Ethics – Data must enable ethical and responsible AI systems, minimising the risk of unintended consequences and harmful outcomes.
- Regulatory compliance – The data being used by AI must be stored, shared, maintained, and used in compliance with legal and regulatory requirements such as GDPR.
Legal checklist
The AI Playbook also features a non-exhaustive summary checklist of legal considerations for the use of AI, drafted by May Winfield, global director of commercial, legal and digital risks at Buro Happold.
She states that before generating AI results or inputting data into a generative AI model, you should ask yourself whether the data is confidential or if client data is being input into a public generative AI.
You should also consider whether the use of the generative AI models is in breach of any confidentiality obligations in contracts, internal policies, or other arrangements.
And you should ask whether there is express copyright for the AI results and/or whether the risks of a copyright breach have been allocated by contract or other method.
May further suggests that quality and/or accuracy checks are carried out before relying on and/or using AI-generated results, and that any internal governance processes or best practice are adhered to.
The age of AI
David Philp, chair of the CIOB’s Digital and Innovation Advisory Panel, was one of the Playbook’s authors. Upon its launch, he said: “Forms of AI are now prevalent in all walks of life and business, and the construction industry is in the age of AI, whether we recognise it or not. Understanding AI in the context of our sector is vitally important to help determine and shape how it might usher in new opportunities.
“AI can immensely support project and construction management, analysing large volumes of project data across the value chain, spotting potential safety risks through computer vision, and offering insights for smarter decision-making. It can also support the automation of repetitive tasks, such as everyday data entry, form filling, and report generation, all of which can dominate a construction manager’s day, so it’s there to be embraced.”
After the launch, BIMplus caught up with Philp.
BIMplus: What did you learn by pulling the Playbook together?
David Philp: The relationship between AI and other aspects of digital construction, especially digital twins – that’s a powerful pairing. AI enhances digital capabilities. We’ve got huge amounts of data now in digital twins, but we need to give that data context so it can support decision-making.
Also, the speed at which AI is developing offers huge innovation opportunities, especially for SMEs.
But we need to be mindful of AI: it’s not the silver bullet. There are various ethical and legal matters to consider, especially EDI. And there’s the black box of decision-making: you put a query in [to the AI], but how does it come to an answer? Is it ethical? How was the AI trained?
But make no mistake, AI and machine learning are really going to reshape our industry. We have to employ this technology to solve the wicked challenges we face – the changes brought on by the Building Safety Act, ageing assets that are often failing, and the hyper object of climate change.
The Playbook reinforces both the role of data and the importance of the quality of data…
100%. At last data is being seen to have strategic value in the built environment. We’re in an age where we’re so reliant on computer-readable data, and we need to continuously improve the data quality: that’s really important to ensure the success of AI and machine learning and other aspects like digital twins.
Good-quality data has got to be the foundation of accurate decision-making.
Organisations need to consider data literacy as a key tenet of what they’re doing as well.
Do organisations need an AI champion or subject matter expert?
Organisations should take a proportionate approach, but having one individual – a bit like some approach BIM – can create a bottleneck. Our recommendation is to form a working group within your organisation focused on developing an AI strategy. That working group should be made up of as many different actors or actresses as possible: not just those in legal and IT, but those at the coalface using the data to make decisions day to day.
You need to understand where you are on your data journey, and you need to be inquisitive.
Don’t miss out on BIM and digital construction news: sign up to receive the BIMplus newsletter.